Ep. 111 Winston Ewert Explains His Research Applying Computer Science to Intelligent Design
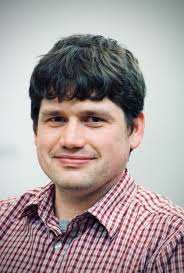
Winston Ewert is an up-and-coming researcher in the Intelligent Design movement, having co-authored articles with one of its leading lights, William Dembski. After explaining ID and relating it to creationism and the theory of common descent, Winston summarizes his research which applies insights from computer science to the biological realm.
Mentioned in the Episode and Other Links of Interest:
- The YouTube video of this interview.
- Biologist H. Allen Orr’s hostile review of Dembski.
- BMS ep 106, in which I discuss my optimistic take on ID’s future.
- Winston Ewert’s article on the dependency graph of life, and his co-authored paper on algorithmic specified complexity.
- William Dembski’s book The Design Inference and his more recent No Free Lunch; and Michael Behe’s book Darwin’s Black Box. #CommissionsEarned (As an Amazon Associate I earn from qualifying purchases.)
- Help support the Bob Murphy Show.
The audio production for this episode was provided by Podsworth Media.
Bob,
Who designed the designer? Just because life is incomprehensible, doesn’t imply there is a creator. It just implies its beyond your comprehension. This just adds an extra step before we get to the point “I can’t comprehend.”
Also, much of the complexity you can’t understand is a manifestation of the structure of the underlying molecules. I’m sure you’re aware of the three body problem. It even gets more difficult to understand when it’s an N-body problem whith N >> 3.
After listening to the entire episode it became clear that Winston (and Bob) can’t see the forest for the tree. They are so wrapped up in the minutia of their arguments that they don’t realize their premise is wrong.
Can you elaborate?
Just because you don’t understand something, doesn’t imply there is a creator. How does an improbable event logically lead to “Creator”? (That’s your false premise) Rather it means you haven’t taken something into account. Or perhaps humans just don’t have the mental capacity to comprehend the complexities of life. Can a squirrel understand string theory? Or perhaps it’s just an error in our thinking: feeling there always needs to be an endless recursion of “why?”.
Adding in God to explain why the world exists doesn’t solve anything. One then needs to explain: where God comes from? It’s just an extra step and you’re still left without the explanation your looking for (except if your religious . Carl Sagan made this arguement in his Cosmos Series.
This idea of “Algorithmic Specified Complexity -> Design” suffers from both false positives and false negatives, and it’s sad that a computer scientist doesn’t see it.
If you were to apply this to encrypted data, it would be indistinguishable from random junk, and you would conclude that it was not designed. You’d be wrong.
And on the other side, since you seem to like the poker example so much, let’s talk about poker. I play (mostly) Texas Holdem. I’ve seen royal flushes. I’ve had 3 royal flushes myself. I’ve seen a royal flush on the board (the community cards). I’ve been dealt AA in Holdem 3 times in a row, which is even less probably than a royal flush. I’ve seen so many other ridiculous things happen, that I can’t even name them all. Should we conclude that this was designed, or the deck rigged, or whatever? Well no, of course not. These cards were shuffled by machines proven in a lab to be random, then cut in a random spot by the dealer. Sometimes the dealer even makes a mistake and accidentally flips a card face up, which alters the deal. So how did these improbable results happen by random chance? Well, simple. There are hundreds of casinos in the world, hosting thousands of poker games, with millions of hands being dealt every day. If somebody, somewhere, didn’t get a royal flush today (assuming casinos weren’t all shut down due to Coronavirus), *that* would be much more suspicious.
And that’s exactly what’s going on in biology. There are quadrillions of living things on the Earth right now. Each of them has billions of base pairs of DNA that split and recombine several times per minute. And this has been going on for about 3 billion years. If you’re going to tell me that seeing a biological royal flush has to be designed because it’s improbable, I’m sorry but that’s not going to cut it. Actual royal flushes in casinos aren’t designed, no matter how improbable they are and how much money you win when you hit one.
Next, to try to push it back to the fitness landscape with NFL theorems just doesn’t make any sense. If your argument is about “specified complexity -> design,” then you need to calculate the specified complexity of the landscape, not say “Well, ok, maybe these specified complex biological constructs aren’t actually designed (which blows the whole theory out of the water, but hey let’s ignore that), but the landscape supports them so that must be designed instead.”
Lastly, as was pointed out on your other pages about ID, none of this is a theory of design, at all. All these guys are doing is trying to knock down known natural processes as capable of producing what we observe. Even if it were successful at doing so, some *unknown* natural process could be responsible for it rather than design. When we ask “what caused Mt. Rushmore to come about,” we don’t sit there and list off geological processes and then put a big X next to each one – no, we say “Look here’s a picture of some humans carving the rock.” *That’s* a theory of design. Who did it, when did they do it, how did they do it? If you aren’t answering these questions, you aren’t proposing a theory at all. You’re just poo-pooing the current best explanations we have.
As a fellow “computer nerd”, I can at least attest that nothing Winston says about Computer Science is wrong or misused (as far as I can tell). I find his pursuit fascinating, and it’s always fun to see CS ideas used in other fields. There are many good ideas that only made sense once we had computers, as Bob mentions in the episode, and they can spill over into other fields.
Here are a couple of thoughts I had while listening:
1.The poker example. Isn’t there something subjective to the Royal Flush being considered valuable? If we played a game of poker where the rules make the Royal Flush score 0, the friend dealing a Royal Flush wouldn’t seem suspicious. It’s not just that all hands have the same probability of occuring, it’s also that our suspicion comes from our friend getting dealt one that is extremely beneficial to him.
2.The firing squad example seems silly. If we had a quasi-infinite number of firing squads, and a tiny percentage (maybe only 1) of prisoners surviving, it wouldn’t seem weird that the one survivor thought he experienced a miracle, or something special. It was probably faulty ammunition or random chance that everybody in the squad missed, or something. To him, it seems miraculous, because this was his only execution (thus far). To us, seeing infinite squads firing and only one (or a small ratio) miss, it seems perfectly reasonable.
3.The context argument is very interesting. I’d say that it seems that the context doesn’t form arbitrarily or randomly, either. For example, the idea of language is to convey ideas. If we lived on a planet where the English letters or English words were naturally, randomly printed onto the ground or rocks, we wouldn’t have developed English – it would be indistinguishable from the background noise, and thus useless to transmit information. The “non-randomness” of a pattern depends on the type of background noise. There’s an idea that we evolved to recognize human faces because it was essential to survival. If human faces were randomly carved into every mountainside on the planet (e.g. natural Mount Rushmores), that ability would be useless. Maybe we wouldn’t have made it, because we’d be unable to tell other people from rocks. Or we would’ve developed more of a focus on voices or smell or whatever.
4.The dependency graph/module idea is very interesting. In my opinion it doesn’t prove design though – why wouldn’t evolution build up a library of modules that depend on each other? Independent of its proving design, I think it’s a great way to model something. If CS is good at something, it’s modeling networks 😀
5.About the XYZ fitness-landscape and algorithms traversing it. The class of algorithms that finds local maxima, as Bob describes, is called “greedy” algorithms (because they go to where they get a higher value and won’t go back). You can tweak them. For example, if you know that your landscape isn’t completely smooth, you can allow the algorithm to explore temporary setbacks. Or if you suspect that it will get trapped in the very first local maximum, you can allow it to venture further out. Of course you have to set limits. Otherwise you end up with an algorithm that explores every possibility, which is usually not feasible, or you would just brute force the optimal solution instead of coming up with an algorithm in the first place. You could argue that Darwinian mutation + natural selection allows for evolution to stray far enough off the local maxima that it could find better ones without explicit intermediate steps. You can also argue that there are intermediate steps that might not seem like such at first glance. E.g. the ability to detect the physical world at all seems an integral part of “life”. Maybe the first “organ” to establish this ability just had part of itself be evaporated by radiation, and then change its behavior due to that. First “detection” module. The next one maybe did heat, or only detected certain other frequencies. Eventually this could be built into audio, visual, and other “detectors”, ending up with an eye.
6.If you’ve ever seen somebody else’s computer code, the argument that designed life wouldn’t have “junk code” in there is absurd. All programmers leave tons of buggy or unused junk code in their programs all the time, no matter how carefully they design their programs.
Oh, I forgot this one:
7.About the “No free lunch” thing. Even if it is true that without specific constraints on the fitness landscape, no algorithm can be proven better than any other, we did end up with a specific fitness landscape. Thus, some algorithm ended up being better than the others. It doesn’t prove that Darwinian evolution would beat all algorithms irrespective of the fitness landscape, but it only needs to work on ours. And in general, random mutation + natural selection seems like a robust enough algorithm that I could see it evolving amongst competing algorithms in many fitness landscapes (so meta!). In contrast, an algorithm that is highly specialized to outperform on a specific fitness landscape would probably fail on most other landscapes, and therefore require knowledge of the underlying landscape constaints. In fact, I can’t think of a better algorithm than random mutation + natural selection if I were given a “veil of ignorance” about the constraints of a fitness landscape, were I to unleash an algorithm upon one.
In my experience with real world engineering problems, it’s rare to find a fitness landscape where gradient following is of no use whatsoever, and genetic algorithms do work quite well … there’s a bunch of work that has been done using generic algorithm optimizers for a huge range of real world problems.
However, there’s a class of problems where fitness landscapes are very carefully chosen to frustrate all known search algorithms and that’s in the sphere of cryptography. What we have discovered is that it’s quite difficult to find a problem that is genuinely so difficult to solve that all attempts are equally as bad as a blind brute force search … but those situations do exist. One example would be the crypto-currency hash algorithm, if there was an easy way to reverse the hash then someone would be out there beating all the other miners.
This begs the question, “Why do we have cryptography anyway?” well because the guy hiding the message is in constant competition with the guy attempting to dig out the hidden message … in other words a competitive environment where only the “fittest” survive and the others are kicked to the side. What I’m getting at here is that the simple gradient follower solves the easy problems, but after those are solved more difficult problems come along. Then smarter approaches solve more of the problems, but that leaves us with even more difficult problems. Most of the interesting problems today are several iterations down the line of this process … so it’s a natural process to move away from easy fitness landscapes towards difficult fitness landscapes.
Hey not-bob, I agree with most of your points, but would like to address a few of them.
“4.The dependency graph/module idea is very interesting. In my opinion it doesn’t prove design though – why wouldn’t evolution build up a library of modules that depend on each other? Independent of its proving design, I think it’s a great way to model something. If CS is good at something, it’s modeling networks”
I don’t think the idea is to necessarily prove design, but rather to disprove descent with modification. Descent with modification wouldn’t produce dependency graphs, because dependencies can jump across relationships. That said, I’m not so sure about how this dependency graph research was actually done. The paper notes that in the hierarchical descent tree, when we see a gene family seem to jump relationships, we assume that those genes were deleted from certain subtrees. Gene deletion is a very real part of biology, and would be undetectable in past lineages, however there are other ways that a gene can disappear from a lineage and still leave evidence behind. For example, primates (including humans) all have a disabled gene that, in its working state, would produce vitamin C internally. This gene wasn’t just lost, it was disabled in a specific manner and was able to be passed on since we receive sufficient vitamin C from our diet. Is this research looking for disabled genes like this, and counting them? Or is it just ignoring such things as they don’t count as “gene families?” It would be nice to know, and the paper doesn’t seem to specify.
> 5.About the XYZ fitness-landscape and algorithms traversing it.
I would say Darwinian evolution *does* in fact find local maxima and get stuck there. That’s why there are so many life forms out there. So why does life continue to evolve? Well, the fitness landscape clearly changes. Not only do geological events happen, but other living things themselves change the landscape, which forces everything else to adapt, etc.
Agreed with both your points.
I wonder, too, if the fitness landscape doesn’t change because of other species using the algorithm at the same time. We’re not talking about 1 species using the algorithm on an empty plane – what if one discovers a local maximum that changes the landscape for itself or other? Think overfishing, or preying on other species. Becomes chaotic very quickly, I imagine (chaotic in the mathematical sense.)
The fitness landscape must be a kind of multi-function. Multiple inputs (all species and environment attributes) and multiple outputs (fitness scores for all species).
There seems to be an assumption here that there is some ultimate purpose behind evolution such that natural selection cannot explain how we got here. However, that is not the case. The only goal in evolution is improving survivability. Natural selection selects those genetic mutations that increased suvivability. This is not a naive search algorithm.
Great episode. Would love to learn more about these concepts.